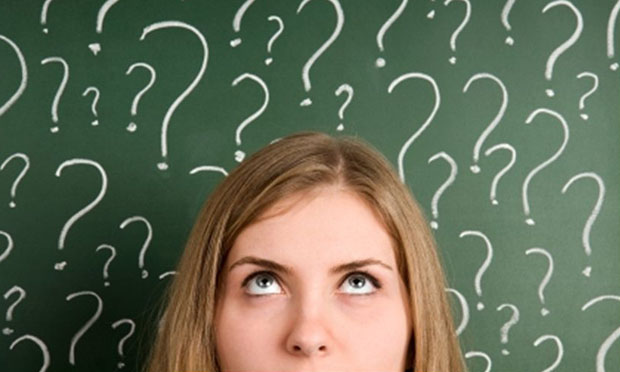
Journalists constantly face the challenge of explaining why things happened: What were the factors in an election victory? What are the reasons behind housing segregation in a city? What is the explanation for a low-performing school? In daily journalism, we are often content to quote relevant sources or officials, and let them do the “explaining.”
But great journalism can do much more than that, particularly if more rigorous thinking and methods are applied. Though journalists need not understand all of the analytical tools of academics, they can benefit from understanding how critical thinking operates in the research world — and using it to their advantage.
There are two reasons why: First, knowing the precise meaning of research-related terms such as “independent variable” or symbols such as “n” can help journalists read and evaluate important studies more effectively. (See our tip sheet on statistical terms for some of the basics, as well as tips on core methods such as regression analysis.) Second, the core journalistic enterprise of verifying information and putting it in context has strong parallels with academic research methods. Both academics and journalists are, in essence, “hypothesis testing”: Data is gathered — statistics, interviews, documents, etc. — and tentative explanations are proposed and tested to arrive at final, defensible explanations of events. Being able to reason in this rigorous way about questions can create deeper, more informed stories.
This type of critical thinking can also benefit the practice of data journalism, where the best work is showing increasing sophistication, but where non-specialists remain at high risk for errors in reasoning and inference.
This overview of academic and critical reasoning comes courtesy of Stephen Van Evera, the Ford International Professor in the MIT Political Science Department. Much of the material in expanded form can be found in his short, useful book Guide to Methods for Students of Political Science. We are grateful to him for allowing us to post an edited version of a memo that was the basis for his work:
I. WHAT IS A THEORY?
Definitions of the term “theory” offered by social science philosophers are cryptic and diverse. The following is a relatively simple framework that captures their main meaning while also spelling out elements often omitted.
Theories are general statements that describe and explain the causes or effects of classes of phenomena. They are composed of causal laws or hypotheses, explanations, and antecedent conditions. Explanations are also composed of causal laws or hypotheses, which are in turn composed of dependent and independent variables. Fourteen definitions are worth mentioning:
1. Law: Laws are observed regular relationships between two phenomena and can be deterministic or probabilistic. The former describe invariate relationships (“If ‘A’ then always ‘B’ “), while the latter frame probabilistic relationships (“If ‘A’ then sometimes ‘B’, with probability ‘X’ “). Hard science has many deterministic laws. Nearly all social science laws are probabilistic. Laws can be causal (” ‘A’ causes ‘B’ “) or spurious (” ‘A’ and ‘B’ are caused by ‘C’; hence ‘A’ and ‘B’ are correlated but do not cause each other”). Our prime search is for causal laws. We explore the possibility that laws are spurious mainly to rule it out, so we can rule in the possibility that observed laws are causal.
2. Hypothesis: A conjectured relationship between two phenomena. Like laws, hypotheses can be causal (“I surmise that ‘A’ causes ‘B’ “) and non-causal (“I surmise that ‘A’ and ‘B’ are caused by ‘C’; hence ‘A’ and ‘B’ are correlated but do not cause each other”).
3. Theory: A causal law (“I have established that ‘A’ causes ‘B’ “) or causal hypothesis (“I surmise that ‘A’ causes ‘B’ “) and an explanation of the causal law or hypothesis that explicates how “A” causes “B.” Note that the term “general theory” is often used for more wide-ranging theories, but all theories are by definition general to some degree.
4. Explanation: The causal laws or hypotheses that connect the cause to the phenomenon being caused, showing how causation occurs. (” ‘A’ causes ‘B’ because ‘A’ causes ‘q’, which causes ‘r’, which causes ‘B’.”)
5. Antecedent condition: A phenomenon whose presence activates or magnifies the action of a causal law or hypothesis. Without it causation operates more weakly (” ‘A’ causes some ‘B’ if ‘C’ is absent, and more ‘B’ if ‘C’ is present”; e.g., “sunshine makes grass grow, but causes large growth in fertilized soil”) or not at all (” ‘A’ causes ‘B’ if ‘C’ is present, otherwise not”; e.g., “sunshine makes grass grow, but only if we also get rain”). An antecedent condition can be restated as a causal law or hypothesis. (” ‘C’ causes ‘B’ if ‘A’ is present, otherwise not”; e.g., “rain makes grass grow, but only if we also get some sunshine”). When referring to antecedent conditions, researchers often use terms such as “interaction terms,” “initial conditions,” “enabling conditions,” “catalytic conditions,” “preconditions,” “activating conditions,” “magnifying conditions,” “assumptions,” “assumed conditions,” or “auxiliary assumptions.”
6. Variable: A concept that can have various values, e.g., the “degree of democracy” in a country or the “share of the two-party vote” for a political party.
7. Independent variable (IV): A variable framing the causal phenomenon of a causal theory or hypothesis. In the hypothesis “literacy causes democracy,” the degree of literacy is the independent variable.
8. Dependent Variable (DV): A variable framing the caused phenomenon of a causal theory or hypothesis. In the hypothesis “literacy causes democracy,” the degree of democracy is the dependent variable.
9. Intervening variables (IntVs): Variables framing intervening phenomena that form a causal theory’s explanation. These phenomena are caused by the independent variable and cause the dependent variable. In the theory “sunshine causes photosynthesis, causing grass to grow,” photosynthesis is the intervening variable.
10. Condition variables (CVs): Variables framing antecedent conditions. Their values govern the size of the impact that IVs or IntVs have on DVs and other IntVs. In the hypothesis “sunshine makes grass grow, but only if we also get some rainfall,” the amount of rainfall is a condition variable.
11. Study variable (SV): A variable whose causes or effects we seek to discover with our research. A project’s study variable can be an IV, DV, IntV, or CV.
12. Prime hypothesis (PH): The overarching hypothesis that frames the relationship between a theory’s independent and dependent variables.
13. Explanatory hypotheses (EH): The intermediate hypotheses that comprise a theory’s explanation.
14. Test hypothesis (TH): The hypothesis we seek to test. Also called the “research hypothesis.” Note that a theory is nothing more than a set of connected causal laws or hypotheses.
II. WHAT IS A SPECIFIC EXPLANATION?
Explanations of specific events (wars, revolutions, elections, economic depressions, etc.) use theories and are framed like theories. A good explanation tells us what specific causes produced a specific phenomenon and identifies the general phenomenon of which this specific cause is an example. Several concepts bear mention:
1. Specific explanation: An explanation cast in specific terms that accounts for a distinctive event. Like a theory it describes and explains cause and effect, but these causes and effects are framed in singular terms. (Thus “expansionism causes aggression, causing war” is a theory; “German expansionism caused German aggression, causing World War II” is a specific explanation.) Specific explanations are also called “particular explanations” (as opposed to a “general explanations”).
2. Non-generalized specific explanation: A specific explanation that does not identify the theory that the operating cause is an example of. For example, the statement “Germany caused World War II” does not answer the question of “Of what is Germany an example?”
3. Generalized specific explanation: A specific explanation that identifies the theories that govern its operation . For example, in the statement “German expansionism caused World War II,” the operating cause, “German expansionism,” is an example of expansionism, which is the independent variable in the hypothesis “expansionism causes war.”
Specific explanations are comprised of four phenomena: “Causal phenomenon” (CP), which are the cause. “Caused phenomenon” (OP) are being brought about by the casual phenomenon. “Intervening phenomena” (IP) are are caused by the causal phenomenon and cause the outcome phenomenon. And finally, “antecedent phenomena” (AP), whose presence activates or magnifies the causal action of the causal and/or explanatory phenomena.
III. WHAT IS A GOOD THEORY?
Seven prime attributes govern a theory’s quality. Good theories:
1. Have large explanatory power: The theory’s independent variable has a large effect on a wide range of phenomena under a wide range of conditions. Three characteristics govern explanatory power:
a. Importance: Does variance in the value on the independent variable cause large or small variance in the value on the dependent variable? An important theory points to a cause that has a large impact, i.e., that causes large variance on the DV. The greater the variance produced, the greater the theory’s explanatory power.
b. Explanatory range: How many classes of phenomena are affected by, hence explained by, variance in the value on the theory’s independent variable? The wider the range of affected phenomena, the greater the theory’s explanatory power. Most social science theories have narrow range but a few gems explain many diverse domains.
c. Applicability: How common is the theory’s cause in the real world? How common are antecedent conditions that activate its operation? The more prevalent the causes and conditions of the theory, the greater its explanatory power. The prevalence of these causes and conditions in the past govern its power to explain history. Their current and future prevalence govern its power to explain present and future events.
2. Elucidate by simplifying: A good theory is parsimonious, using few variables simply arranged to explain its effects. However, parsimony often requires some sacrifice of explanatory power. If that sacrifice is too large it becomes unworthwhile. We can tolerate some complexity if we need it to explain the world.
3. Are “satisfying”: A good theory satisfies our curiosity and an unsatisfying one leaves us wondering what causes the cause proposed by the theory. A politician once explained her election loss: “I didn’t get enough votes!” This is true but unsatisfying. We still want to know why she didn’t get enough votes. The farther removed a cause stands from its proposed effect, the more satisfying the theory. Thus “droughts cause famine” is less satisfying than “changes in ocean surface temperature cause shifts in atmospheric wind patterns, causing shifts in areas of heavy rainfall, causing droughts, causing famine.”
4. Are clearly framed: A clearly framed theory includes a full outline of the theory’s explanation and does not leave us wondering how “A” causes “B.” Thus “changes in ocean temperature cause famine” is less complete than “changes in ocean temperature cause shifts in atmospheric wind patterns, causing shifts in areas of heavy rainfall, causing droughts, causing famine.”
A clearly framed theory includes a statement of the antecedent conditions that enable its operation and govern its impact. Otherwise we cannot tell what cases the theory governs, and thus cannot infer useful policy prescriptions. Foreign policy disasters often happen because policymakers apply valid theories to inappropriate circumstances. Consider the hypothesis that “appeasing other states makes them more aggressive, causing war.” This was true with Germany during 1938-1939, but the opposite is sometimes true: A firm stand can make the other more aggressive, causing war. To avoid policy backfires, policymakers must know the antecedent conditions that decide if a firm stand makes others more or less aggressive. Parallel problems arise in all policymaking domains and highlight the importance of framing antecedent conditions clearly.
5. Are in principle “falsifiable”: Theories that are not clearly framed may prevent investigators from inferring predictions from them. Theories that make omni-predictions that are fulfilled by all observed events also are non-falsifiable. Empirical tests cannot corroborate or infirm such theories because all evidence is consistent with them. Religious theories of phenomena have this quality: happy outcomes are God’s reward, disasters are God’s punishment, cruelties are God’s tests of our faith, and outcomes that elude these broad categories are God’s mysteries.
6. Explain important phenomena: A good theory answers questions that matter to the wider world, or it helps others answer such questions. Theories that answer unasked questions are less useful even if they answer these questions well. (Much social science theorizing has little real-world relevance.)
7. Have prescriptive richness: A good theory yields useful policy recommendations. A theory gains prescriptive richness by pointing to manipulable causes, since manipulable causes might be controlled by human action. Thus “capitalism causes imperialism, causing war” is less useful than “offensive military postures and doctrines cause war,” even if both theories are equally valid, because the structure of national economies is less manipulable than national military postures and doctrines. “Teaching chauvinist history in school causes war” is even more useful, since the content of national education is more easily adjusted than national military policy. A theory gains prescriptive richness by identifying dangers that could, with warning, be defeated or mitigated by timely countermeasures. Thus theories explaining the causes of hurricanes provide no way to prevent them, but they do help forecasters warn threatened communities.
IV. HOW CAN THEORIES BE MADE?
There is no agreed recipe for making theories. Some scholars use deduction, inferring explanations from more general, already-established causal laws. Thus much economic theory is deduced from the assumption that people seek to maximize their personal economic utility. Others make theories inductively: they look for relationships between phenomena; then they investigate to see if discovered relationships are causal; then they ask “of what more general causal law is this specific cause-effect process an example?” For example, after observing that clashing efforts to gain secure borders helped cause the Arab-Israeli wars, a theorist might suggest that competition for security causes war.
Nine aids to theory-making bear mention. (The first eight are inductive methods, the last is deductive.)
1. Examine “outliers”: Cases that are poorly explained by existing theories may have some unknown cause. To make a new theory we select cases where the phenomenon we seek to explain is abundant but its known causes are scarce or absent. Unknown causes will announce themselves as unusual characteristics of the case, and as phenomena that are associated with the dependent variable within the case. We also cull the views of people who experienced the case or know it well and nominate their explanations as candidate causes. To infer a theory’s antecedent conditions (CVs) we select cases where the DV’s causes are abundant but the DV is scarce or absent. This suggests that unknown antecedent conditions are absent in the case.
2. “Method of difference” and “method of agreement”: In the first, the analyst compares cases with similar background characteristics and different values on the study variable (i.e., the variable whose causes or effects we seek to discover), looking for other differences between cases. These other cross-case differences are nominated as possible causes of the study variable (if we seek to discover its causes) or possible effects (if we seek its effects). Similar cases are picked to reduce the number of candidate causes or effects that emerge: more similar cases produce fewer candidates, making real causes and effects easier to spot. In the method of agreement, the analyst explores cases with different characteristics and similar values on the study variable, looking for other similarities between the cases, and nominating these similarities as possible causes or effects of the variable.
3. Select cases with high or low study variable (SV) values: If values on the SV are very high (i.e., the SV phenomenon is present in abundance) its causes and effects should also be present in unusual abundance, standing out against the case background. If values on the SV are very low (i.e., the SV phenomenon is absent) its causes and effects should also be prominent by their absence.
4. Select cases with extreme within-case variance in the study variable: If values on the SV vary sharply, phenomena that co-vary with the SV should also vary sharply, standing out against the more static case background.
5. Counterfactual analysis: The analyst examines history, trying to “predict” how events would have unfolded had a few elements of the story been changed, with a focus on varying conditions that seem important and/or manipulable. For instance, to explore the effects of military factors on the likelihood of war, one might ask: “How would pre-1914 diplomacy have evolved if the leaders of Europe had not believed that conquest was easy?” Or, to explore the importance of broad social and political factors in causing Nazi aggression: “How might the 1930s have unfolded had Hitler died in 1932?” The greater the changes that one’s analysis suggests would have followed from the changes posited, the more important one’s analysis. When analysts discover counterfactual analyses they find persuasive, they have found theories they find persuasive, since all counterfactual predictions rests on theories.
6. Infer theories based on policy debates: Proponents of given policies frame specific cause-effect statements (“If communism triumphs in Vietnam, it will triumph in Thailand, Malaysia and elsewhere”) that can be framed as general theories (“Communist victories are contagious: communist victory in one state raises the odds on communist victory in others”) that can be tested. Such tests in turn can help resolve the policy debate. Theories inferred in this fashion are sure to have policy relevance and they merit close attention.
7. Seek insights from actors or observers: Those who experience an event often observe important data that are unrecorded and thus lost to later investigators. Hence they can suggest hypotheses that could not be inferred from direct observation alone.
8. Explore large-n data sets: Discovered correlations are nominated as possible cause-effect relationships. This method is seldom fruitful, however. A new large-n data set is usually hard to assemble, but if we rely on existing data sets our purview is narrowed by the curiosities of previous researchers. We can only explore theories that use variables that others have already chosen to code.
9. Adapt theories from another domain: Students of misperception in international relations and students of mass political behavior have both borrowed theories from psychology. Students of military affairs have borrowed theories from the study of organizations. Students of international systems have borrowed theories (e.g., oligopoly theory) from economics.
V. HOW CAN THEORIES BE TESTED?
There are two basic ways to test theories: experimentation and observation. Observational tests come in two varieties: large-n and case study. Thus, overall we have a universe of three basic testing methods: experimentation, observation using large-n analysis, and observation using case study analysis.
1. Experimentation: An investigator infers predictions from a theory. Then the investigator exposes one of two equivalent groups to a stimulus while not exposing the other group. Are results congruent or incongruent with the predictions? Congruence of prediction and result corroborates the theory, incongruence infirms it.
2. Observation: An investigator infers predictions from a theory, then observes the data without imposing an external stimulus on the situation, and asks if observations are consistent with predictions. Two types of observational analysis can be performed:
a. Large-n, or “statistical,” analysis: A large number of cases — usually several dozen or more — is assembled and explored to see if variables shift as the theory predicts.
b. Case study analysis: A small number of cases (as few as one) are explored in detail, to see if events unfold in the manner predicted and (if the subject involves human behavior) if actors speak and act as the theory predicts.
Which method — experiment, observation large-n, or observation case study — is best? Some hard sciences (chemistry, biology, physics) rely largely on experiments. Others (astronomy, geology, paleontology) rely largely on observation.
In political science experiments are seldom feasible, with rare exceptions (e.g. conflict simulations or psychology experiments), leaving observation as the prime method of testing. Large-n methods are relatively effective for testing theories of American electoral politics because very large numbers of cases (of elections, or of interviewed voters) are well-recorded. Case studies can be strong tools for exploring American politics, especially if in-depth case studies yield important data that is otherwise inaccessible.
VI. STRONG VS. WEAK TESTS; PREDICTIONS AND TESTS
Strong tests are preferred because they convey more information and carry more weight than weak tests. A strong test is one whose outcome is unlikely to result from any factors except the operation or failure of the theory. Strong tests evaluate predictions that are certain and unique: A certain prediction is an unequivocal forecast, and the more certain, the stronger the test. A unique prediction is a forecast not made by other known theories, and the more unique, the stronger the test. The most unique predictions forecast outcomes that could have no plausible cause except the theory’s action.
Certainty and uniqueness are both matters of degree. Tests of predictions that are highly certain and highly unique are strongest, since they provide decisive positive and negative evidence. As the degree of certitude or uniqueness falls, the strength of the test also falls. Tests of predictions that have little certitude or uniqueness are weakest, and are worthless if the tested prediction has no certitude or uniqueness.
There are four types of tests, differing by their combinations of strength and weakness:
1. Hoop tests. Predictions of high certitude and no uniqueness provide decisive negative tests: a flunked test kills a theory or explanation, but a passed test gives it little support. For example: “Was the accused in town on the day of the murder?” If not, he’s innocent, but showing that he was in town does not prove him guilty. To remain viable the theory must jump through the hoop this test presents, but passage of the test still leaves the theory in limbo.
2. Smoking gun tests. Predictions of high uniqueness and no certitude provide decisive positive tests: passage strongly corroborates the explanation, but a flunk infirms it very little. For example, a smoking gun seen in a suspect’s hand moments after a shooting is relatively conclusive proof of guilt, but suspects not seen with a smoking gun are not proven innocent. An explanation passing a test of this sort is strongly corroborated, but little doubt is cast on an explanation that fails the test.
3. Doubly-decisive tests. Predictions of high uniqueness and high certitude provide tests that are decisive both ways: passage strongly corroborates an explanation, a flunk kills it. If a bank security camera records the faces of bank robbers, its film is decisive both ways — it proves suspects guilty or innocent. Such tests combine both a “hoop test” and “smoking gun test” and convey the most information, but are rare.
4. Straw-in-the-wind tests. Most predictions have low uniqueness and low certitude are indecisive both ways: Passed and flunked tests provide straws in the wind but are themselves indecisive. Thus many explanations for historical events make probabilistic predictions (“If Hitler ordered the Holocaust, we should probably find some written record of his orders”), whose failure may simply reflect the downside probabilities. We learn something by testing such straw-in-the-wind predictions, but such tests are never decisive by themselves. Unfortunately, this describes the predictions we usually work with.
Strong tests are preferred to weak tests, but tests can also be hyper-strong, i.e., unfair to the theory. For example, one can perform tests under conditions where countervailing forces are present that counteract its predicted action. Passage of such tests is impressive because it shows the theory’s cause has large importance, i.e., high impact. However, a valid theory may flunk such tests because the countervailing factor masks its action. Such a test misleads by recording a false negative — unless the investigator, mindful of the test’s bias, gives the theory bonus points for the extra hardship it faces.
VIII. HOW CAN SPECIFIC EVENTS BE EXPLAINED?
Ideas framing cause and effect come in two broad types: theories and specific explanations. Theories are cast in general terms and could apply to more than one case (e.g., “expansionism causes war,” or “impacts by extraterrestrial objects cause mass extinctions”). Specific explanations explain discrete events — particular wars, interventions, empires, revolutions, or other single occurrences (e.g., “German expansionism caused World War II,” or “an asteroid impact caused the extinction of the dinosaurs”). The framing and testing of theories is covered above, but how should we evaluate specific explanations? Four questions should be asked:
1. Does the explanation exemplify a valid general theory? To assess the hypothesis that “a” caused “b” in a specific instance, we first assess the hypothesis’ general form (” ‘A’ causes ‘B’ “). If “A” does not cause “B,” we can rule out all explanations of specific instances of “B” that assert that examples of “A” were the cause, including the hypothesis that ‘a’ caused ‘b’ in this case. The argument that “the rooster’s crows caused today’s sunrise” is assessed by asking whether, in general, roosters cause sunrises by their crowing. If the hypothesis that “rooster crows cause sunrises” has been tested and flunked, we can infer that the rooster’s crow cannot explain today’s sunrise. The explanation fails because the covering law is false.
Generalized specific explanations are preferred to non-generalized specific explanations because we can measure the conformity of the former but not the latter with their covering laws. (The latter leave us with no identified covering laws to evaluate.) Non-generalized specific explanations must be re-cast as generalized specific explanations before we can measure this conformity.
2. Is causal phenomenon present in the case we seek to explain? A specific explanation is plausible only if the value on the independent variable of the general theory on which the explanation rests is greater than zero. Even if “A” is a confirmed cause of “B,” it cannot explain instances of “B” that occur when “A” is absent. Even if economic depressions have been shown to cause war, this theory does not explain wars that occur in periods of prosperity. Asteroid impacts may cause extinctions, but cannot explain extinctions that occurred in the absence of an impact.
3. Are the covering law’s antecedent conditions met in the case? Theories cannot explain outcomes in cases that omit their necessary antecedent conditions. Dog bites spread rabies if the dog is rabid; bites by a non-rabid dog cannot explain a rabies case.
4. Are the covering law’s intervening phenomena observed in the case? Phenomena that link the covering law’s posited cause and effect should be evident and appear in appropriate times and places. Thus if an asteroid impact killed the dinosaurs 65 million years ago we should find evidence of the catastrophic killing process that an impact would unleash. For example, some theorize that an impact would kill by spraying the globe with molten rock, triggering global forest fires that darken the skies with smoke, shutting out sunlight and freezing the earth. If so, the soot these fires would generate should be found in 65-million year old sediment worldwide. We should also find evidence of a very large (continent-sized or even global) molten rock shower, and of a very abrupt dying of species.
This fourth step is necessary because the first three steps are not definitive. If we omit step four it remains possible that the covering law that supports our explanation is probabilistic and the case at hand is among those where it did not operate. We also should test the explanation’s within-case predictions as a hedge against the possibility that our faith in the covering law is misplaced, and that the “law” is in fact false. For these two reasons, the better the details of the case conform to the detailed within-case predictions of the explanation the stronger the inference that the explanation explains the case.
Analysts are allowed to infer the covering law that underlies the specific explanation of a given event from the event itself. The details of the event suggest a specific explanation; that explanation is then framed in general terms that allow tests against a broader database; these tests are passed; and the theory is then re-applied to the specific case. Thus general theory-testing and specific case-explaining can be done together and can support each other.
Tags: training